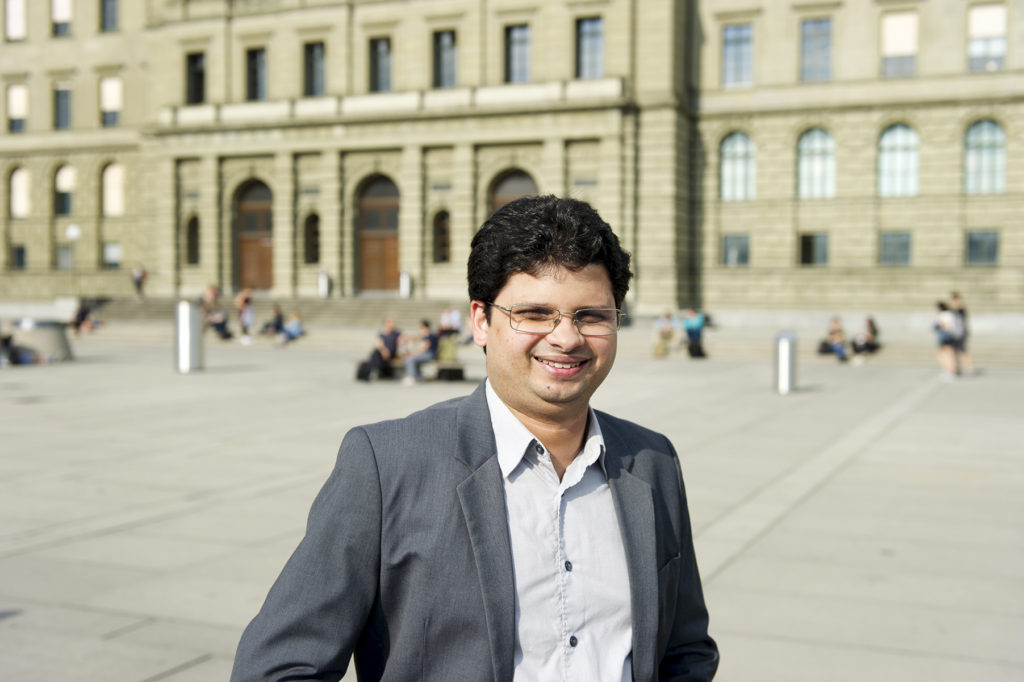
Siddhartha Mishra is a Professor in the Department of Mathematics at Eidgenössische Technische Hochschule (ETH), Zurich. He is an expert in the design and analysis of provably efficient numerical algorithms for nonlinear partial differential equations, which he then validates on high-performance computing platforms. Winner of the 2019 Infosys Science Prize for Mathematics, Siddhartha spoke extensively with Bhāvanā, fondly remembering his student days spent in contemplation under the tall, nurturing trees of the idyllic campus of the Indian Institute of Science.
Professor Siddhartha Mishra: Thank you for taking time out to speak with us. We greatly appreciate your warm gesture. Let us start by first congratulating you on winning the prestigious Infosys Prize for Mathematical Sciences, for the year 2019.
SM: Thank you very much.
Can you please tell us a little bit about your early years, starting from your childhood, and also dwelling on your family background?
SM: I was born in Bhubaneswar (the capital of the state of Odisha) in a middle-class family. My father, Rabi Prasan Mishra was the general manager of a hotel, run by the state tourism development corporation at that time. He later worked as a Tourism promotion officer, in Bhubaneswar and Puri (a beach and pilgrim town near Bhubaneswar). I grew up in both places. My mother, Rashmi Mishra, was the primary caregiver and teacher for both my brother and me in our early years. They came from middle-class families with professionals such as doctors, teachers and bureaucrats on both sides. I attended primary school in Bhubaneswar and secondary school in Puri, before returning to Bhubaneswar for higher secondary school (11th and 12th grades). My paternal grandfather, Gopinath Mishra, was a retired bureaucrat and was the main influence in my early years. He had a wealth of life experience, was fluent in many languages, and was an excellent storyteller. My interest in politics and sports (especially cricket and football) can be attributed to him.
As a young boy growing up in Orissa, as it was called then, do you recall any significant event that may have triggered off your early love for mathematics, or perhaps in a broader sense, maybe even for the sciences in general?
SM: It is interesting that you ask this question. To be honest, I had no specific interest in science or mathematics in my primary school years. I grew up in campuses of hotels, where a lot of people, including many from outside India, came and stayed. So, in my early years, my interests lay in interacting with people from different countries, identifying these countries on a map and quizzing my grandfather about them. I was certainly more interested in geography and sports than in mathematics. I was reasonably good at mathematics in primary school but not outstanding, to the best of my memory. However, things changed when I started secondary school. I got more interested in physics and mathematics around my 7th grade, and there was a kindling of interest in understanding how things worked.
Did you have a strong reading habit as a youngster growing up in Bhubaneshwar and Puri? If so, what in particular held your interest? Or, was your choice eclectic?
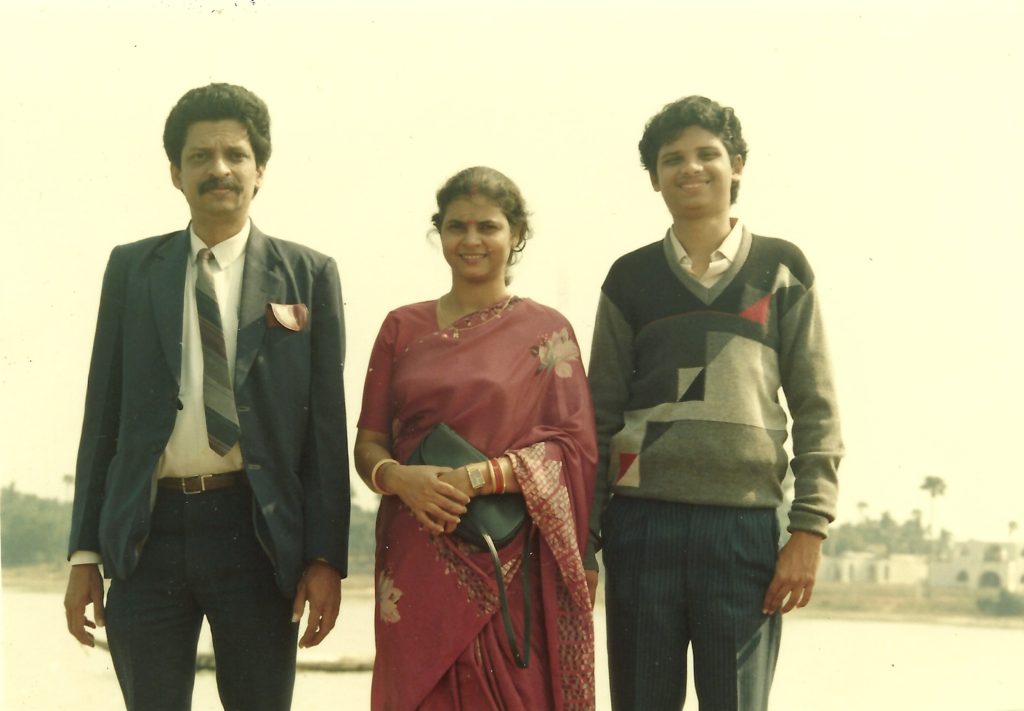
SM: I read a lot as a child and still keep on doing so. In the early years, it was mostly children’s literature, children’s editions of novels and adventure books. I also read a lot of newspapers and magazines. We used to subscribe to The Statesman and The Telegraph (Kolkata-based newspapers that are popular in Eastern India) and to the India Today magazine, and I used to read all of them regularly, and certainly by the age of ten. I had not read any science books yet, but that changed when we started subscribing to a magazine called Science Reporter. It had a lot of content on popular science, and this magazine certainly kindled my interest. There was later on a stage where I would read anything that I could lay my eyes on. I still read quite a bit of fiction and non-fiction, which has nothing to do with science, let alone with mathematics.
On a related note, have you followed the rich literary heritage of the Odia language? If so, whose writings have influenced you?
SM: Unfortunately, my abilities with the Odia language are a bit limited. I speak, read and understand Odia very well, but my fluency with literary Odia is low. In particular, I have rarely read Odia literature outside of my school curriculum, and even this was a long time back. My limited Odia repertoire is something that I am a bit ashamed of, and which I would like to rectify at some point of time. Among my ancestors, Odia was indeed the spoken language, but I do not recall any of them coming up with a substantial body of written work in Odia. On the other hand, many of them on both sides of the family tree, were educated in Sanskrit and may have probably even written in that language too, although I am unable to locate their writings.
Most people in the sciences identify at least one strong positive influence in their formative years, who would have eventually gone on to play a decisive role in shaping the young person’s early impressions of the world. Is there somebody who played that role in your own life?
SM: It is difficult to pinpoint a single dominant influence. However, when I was in secondary school, I had a very good teacher named Umakant Mishra. He was a brilliant mathematics and physics teacher who first exposed me to the beauty and complexity of mathematics. He certainly played a big role in awakening my interest in mathematics and physics early on. Other science teachers in school also played their part.
Were you good in extracurricular activities as well? For instance, did debating, or even perhaps quizzing, catch your fancy then as a young student?
SM: I was a very good quizzer in my school and college days, winning quizzes in school, college, as well as at the state level. I still have an interest in quizzing. I was also a good debater, and excelled at writing essays in both school and college. I won many prizes, both individually and as part of a team. I also liked sports but did not have much ability, or success in competitive sports.
Where did your high school education happen?
SM: I studied at Blessed Sacrament High School in Puri from the 8th to 10th grades. For the 11th and 12th grades, I studied at Buxi Jagabandhu Bidyadhar (BJB) Autonomous College in Bhubaneswar. As in many states of India, one has to specialize in the 11th and 12th grades, and so I studied mathematics, physics, chemistry and electronics.
You later joined Utkal University for your undergraduate education. What subjects did you study then?
SM: I continued to study at BJB College for my bachelor’s degree, which was awarded by Utkal University. I studied both physics and mathematics for my bachelor’s degree. Statistics was also a minor subject.
Also by then, had the seed of the idea of a future career in research already begun to take root in your mind?
SM: Yes, absolutely. I had already resolved in high school that I wanted to be a researcher, hopefully, a theoretical physicist. When I was in the 10th grade, I was fascinated after reading A Brief History of Time by Stephen Hawking. It got me interested in cosmology and astrophysics. I also read books by Roger Penrose around the same time. In high school, I started reading quite a lot of advanced material, mostly on physics. So much so, that I probably did not concentrate on the syllabus as much as I should have. This interest in physics was further strengthened in college. I spent a lot of time in the college library, reading books on gravitation and quantum mechanics. So, the decision to do what it takes to become a professional scientist was already made around the time I finished high school.
Peer influence, if not peer pressure, is a major component of important decisions that most young people eventually take, especially so while being in the early part of their careers. Did your peer group also have a bearing on your decision to study mathematics?
SM: You ask an interesting question. In this case, peer influence had the opposite effect on me. As you know quite well, there is an enormous amount of societal and peer pressure in India to study engineering or medicine after high school. I too felt the same pressure. However, I had resolved to resist it. I had no interest in medicine whatsoever. I also did not see myself as a professional engineer. So, I did not write the engineering entrance exams with any serious preparation. Everyone else in my peer group in high school was preoccupied with writing the entrance exams, and almost all of them went on to study engineering. I was the sole exception. This trend continued during my college years. All of my friends who graduated in engineering, and also those who studied the sciences, were also preparing either for entrance exams for management studies, or for the civil services. Hence, my college years were a very lonely time in this respect. But my parents and friends were very supportive of my choice, I’m happy to admit.
You chose to study mathematics at the Indian Institute of Science (IISc), having enrolled in a program run jointly by IISc and the Tata Institute of Fundamental Research (TIFR) then. Did you consider any other places, or perhaps even subjects other than mathematics at that point in time?
SM: After I finished the requirements for my bachelor’s degree, I was looking at programmes offering a PhD in either mathematics or physics. IISc had just then extended its integrated PhD program to include mathematical sciences, and this seemed like an attractive option. I had also applied for the integrated PhD program in physics at IISc but was not allowed to write the entrance test in two subjects. So, I wrote the mathematics entrance exam. Therefore, my initiation into higher studies in mathematics was a bit accidental.
![]() |
![]() |
We learn that you even had a small stint working with the Bhubaneswar chapter of an English daily before you joined IISc. How did this come about? Are there any interesting experiences from that stint that you now fondly recollect?
SM: It was for a publication called The Asian Age, and it was just for a few days of internship, essentially to observe how a newspaper works. I very quickly realized that I was not cut out to be a journalist. The whole experience was one driven by curiosity, but it ended quickly.
The program you enrolled for at IISc was then a joint program between IISc and TIFR. Tell us a little bit about this joint program, and also on how it nurtured young students like you, helping you to blossom into world-class researchers in mathematics?
SM: As I mentioned earlier, IISc had an Integrated PhD program (MS + PhD) for the other sciences and they started one for mathematics too in 2000, which is when I enrolled as one of the students of the very first batch. The TIFR Centre had been functioning from within IISc campus since the early seventies. It was started by Prof. K.G. Ramanathan from TIFR Mumbai, to create the intellectual infrastructure required for high-quality research in Applied Mathematics in India. The IISc campus was an excellent location as it allowed close interaction between the TIFR Centre and different engineering departments of IISc. There was a thriving joint IISc-TIFR program that lasted from the early 1970s to the end of the 1980s, but was discontinued thereafter. The program was again reborn in the form of the Integrated PhD program in the year 2000, and I really benefited from it. The academic environment was almost ideal. IISc’s campus offers a very high quality of life, and the synergies of the strengths of the mathematics department at IISc, and the core competencies of TIFR Centre led to a very dynamic program. Moreover, it was possible for me to take courses offered by other departments at IISc, and I availed of this opportunity to attend courses in computer science, physics and aerospace engineering.
Was the course work at IISc-TIFR very different from the comparatively rather pedestrian course work usually seen in most other conventional Indian universities? Did you also consequently have to dig into your inner reserves in order to compete and excel, in what must have been a very new, and a potentially highly challenging environment?
SM: The course work was very different from what was taught at a conventional university. In the first year alone, we had ten core courses, which more or less corresponds to the full course work, over two years, at a conventional university. With five courses a semester, the schedule was very hectic. Besides, one had to maintain a minimum grade point average to continue the program–-so the heat was always on. In the second year, all the courses were electives. So, there was the option of specializing in one’s chosen direction very quickly. This is exactly what I did. Almost all of my courses in the second year were in analysis, partial differential equations (PDEs) and related areas.
The transition between college and IISc-TIFR was a real shock to me. The intellectual requirements and the sheer effort needed to do well were orders of magnitude higher in comparison to most other Indian universities. So, I have to confess that it was difficult at times, particularly so in the first year. It required a considerable amount of support from my teachers, especially Profs. Adimurthi and M. Vanninathan (TIFR Centre). The help I received from my seniors, especially from Profs. Prashanth Srinivasan (then a postdoc at TIFR) and Sandeep K (then finishing his PhD at TIFR), was critical in me being able to handle the tough situation. I will always remember their contributions at that crucial juncture.
Even before you arrived at IISc, were you ever a participant in the highly successful pedagogy experiment that Prof. Kumaresan and his team of enthusiastic colleagues in the Mathematics Training and Talent Search (MTTS) Programme have put together–-to train underprepared undergraduate students from conventional universities, for eventual research and teaching careers? If so, what are your impressions of the program?
SM: Unfortunately, I never attended the MTTS program. I had applied for it in 1999 but was not selected. After I joined IISc-TIFR, I came to know of the program in great detail. I had classmates and colleagues who had attended the program, and who also knew faculty who taught at the program. I think Prof. Kumaresan’s efforts are commendable. It is well known that the difference in academic levels, between elite institutions such as TIFR and IISc on the one hand, and conventional universities on the other, is vast. There is a pressing need to bridge this gap and thus provide talented and motivated students at universities the opportunity to access globally benchmarked education; and MTTS fills many parts of this gap. Also, the solution to this serious problem would have to be twofold–-one is to increase the number of globally benchmarked institutions in India which can admit well-prepared minds, and to also simultaneously raise the standards of pedagogy in conventional universities. The fairly recent founding of seven Indian Institute of Science Education and Research (IISER) campuses, along with an increase in the number of the Indian Institute of Technology (IITs) addresses a part of the problem.
The difference between pure and applied mathematics is the time horizon of applicability
Did subjects in pure mathematics, say number theory or algebraic geometry, ever appeal to you as a potential research path? Also, do you feel that there really is a concrete difference between pure and applied mathematics?
SM: I will start with the latter question first. The differences between pure and applied mathematics, as the two are conventionally delineated, are fairly minor. Both fields of study use rigorous mathematical techniques to solve problems, specifically in their emphasis on rigorous proofs. The difference, in my opinion, is what I call the time horizon of applicability. The results of a pure mathematician may or may not be applied in another discipline (say, outside of mathematics) and even if they are, this might, on an average, take a long time as it requires hitherto unknown facts to first emerge, and then consolidate. For instance, people have been studying prime numbers for a long time, but it is only fairly recently that they are being used in cryptography. Clearly, people studying properties of prime numbers at the beginning of the 20th century did not envisage their use in cryptography fifty to seventy five years later.
On the other hand, the results of an applied mathematician might be used the very next day in other sciences, and also in industry. In any case, the time horizon here is much shorter, and the applied mathematician is aware of this fact, and therefore consciously premises his/her research on this distinct possibility. As an example, look back on how quickly wavelets came to be used in signal processing, and computer vision. This to me is the main difference between so-called pure and applied mathematics.
Coming to your first question–-once I had finished my first year of taking the mandatory courses at TIFR Centre, I knew that I had grown to become very interested in PDEs, perhaps also owing to my early interest in physics. So, there was never any desire to do research in other areas of mathematics. Nevertheless, I did attend courses in subjects such as Algebraic Topology, and Geometry during my studies.
Let us get to the actual research work that you have been involved in, for the last fifteen years at least, which is the numerical analysis of partial differential equations. Give us an idea about the nature of the broad theme of your work.
SM: The key, broad theme of my work is the design, analysis and implementation of robust and efficient algorithms for simulating complex systems that arise in science and engineering, on high-performance computing (HPC) platforms. As many of these systems are modelled by PDEs, the focus of my research group is on efficient algorithms for simulating PDEs. As you know quite well, it is not possible to write down explicit solution formulae for most PDEs, so numerical simulation is both inevitable and essential.
PDEs come in different varieties and one has to focus on specific types. I have concentrated on nonlinear hyperbolic, and related PDEs over the last 15 years. These arise in a wide variety of applications, for instance in astrophysics, geophysics, climate science, aerospace and mechanical engineering, among others. However, I have also worked on other types of PDEs, particularly of the parabolic type for other applications.
So you are saying that naturally occurring phenomena can be modelled using these continuous objects called PDEs; and also, that these continuous objects would further need to be “discretized’’, so as to set them up and solve them on digital computers. Does anything significant transpire during the process of discretization, which people would therefore need to be acutely mindful of? In essence, what are the hazards of not understanding the consequences of discretization?
SM: Yes, PDEs are continuous objects. However, computers can only handle discrete (finite) objects as inputs and provide discrete objects as outputs. So, one has to discretize the PDE in order to be able to use a computer to approximate it. Discretization is a process of approximation. Whenever any object is approximated, it is essential to ascertain the quality of approximation by computing the so-called `error’ i.e., the difference between the ground truth (the underlying solution of the PDE) and the approximation (discretized version of the same). Obviously, this error (measured in a suitable norm or distance) depends on many things, most importantly on the number of discretization points, also called degrees of freedom in the literature.
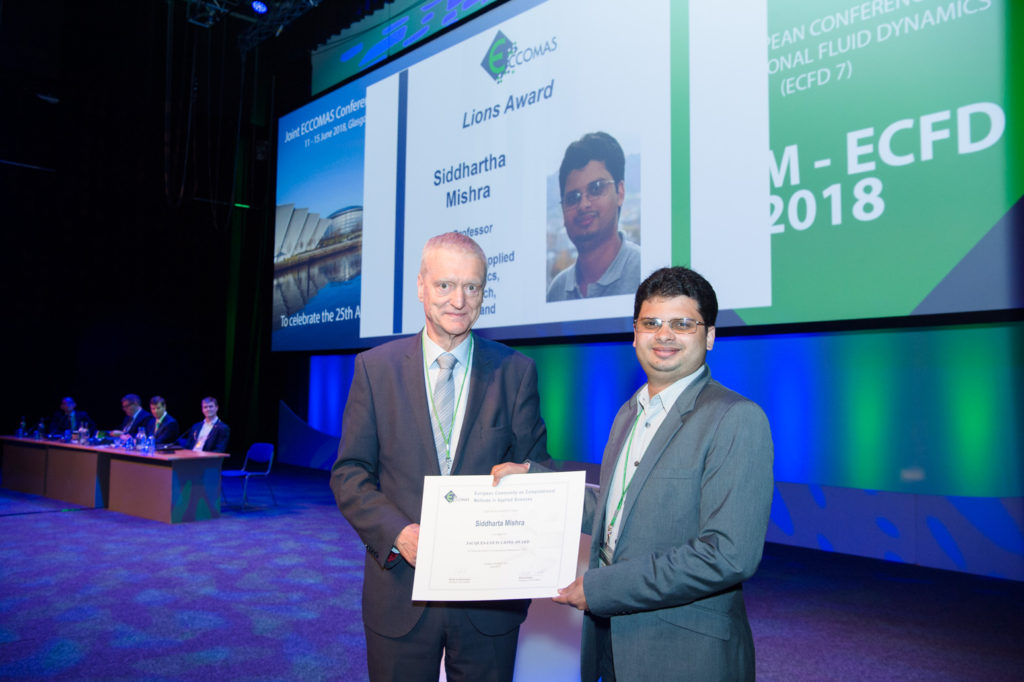
So, a key question in numerical analysis is to estimate this discretization error. In particular, the error should decrease as the number of degrees of freedom increases. Hence, in the limit of an infinite number of degrees of freedom, we say that the approximation converges to the underlying solution of the PDE (the ground truth). It is absolutely essential to establish convergence for a numerical method. Otherwise, there is no guarantee that the numerical method is going to approximate the PDE well. In fact, in numerical analysis, it is also equally important to determine the so-called rate of convergence, which tells us the speed at which the error decreases as the number of degrees of freedom are increased. This rate enables us to estimate the quality of the approximation, in particular, the computational cost which has to be necessarily expended in order to attain a certain acceptable/desired level of error. Practitioners need this information in order to rationally allocate their computational resources. Let us choose to call an algorithm `efficient’ if it is able to attain low errors at a reasonably low computational cost. A pressing concern in numerical analysis is the design of such efficient algorithms.
The long history of numerical analysis has taught us that a first step towards the design of convergent and efficient algorithms is to ensure that they are also stable. This already goes back to Peter Lax, and arguably, even earlier to John von Neumann and possibly all the way up to Richard Courant, Kurt Friedrichs and Hans Lewy. Stability of an algorithm provides the ironclad guarantee that the output of the algorithm will remain within a reasonable range, itself dictated by the underlying PDE. Outputs outside this range are treated as gibberish. So, when we start the process of designing an algorithm, we first ensure that it is stable, in a suitable sense. Then, we try to provide rigorous arguments about convergence and finally, we estimate the error in terms of the number of degrees of freedom (accuracy), and the computational cost, again in terms of the number of degrees of freedom. The keywords in the above process are stability (robustness), convergence, accuracy and efficiency.
The governing equations of fluid dynamics have for long held your attention. How ubiquitous are their conservation laws in terms of their relevance to researchers studying diverse phenomena–-ranging from climate modelling to aerodynamics, or even from solar physics to avalanches and tsunamis?
SM: They indeed are ubiquitous. Models in physics are either based on variational principles (wherein some energy or action has to be minimized, in order to determine the state of the system), or conservation laws (involving quantities of interest that are conserved by the system during its evolution). Hence, there are a large number of physical phenomena that are described by conservation laws. The specific systems that you ask about are very pertinent. It is exciting to realize that the same (or a very similar) set of equations can describe manifestly diverse phenomena, and that too, over completely different scales. After all, one begins by imposing the conservations of mass, momentum and energy. Just this much is sufficient to derive the governing equations of climate dynamics (anelastic Euler or Navier–Stokes equations), of solar physics via the equations of MagnetoHydroDynamics (MHD), of aerodynamics (compressible Euler and Navier–Stokes equations), and even the propagation of tsunamis and avalanches (shallow-water type models). It is quite remarkable to realize that so many diverse physical phenomena can be expressed in terms of only a few, basic governing equations.
Truly remarkable. Why is the notion of `nonlinearity’ such a critical idea while studying PDEs in general, and what typically are its key consequences?
SM: Nonlinearity is best encapsulated in how a system responds to inputs. For a linear system, a small change in the input would lead to a proportionately small change in the output, and similarly too for large changes. On the other hand, for a nonlinear system, a small change in the input might lead to a very large change in the output, and vice versa. The most famous example is provided by the well-known butterfly effect. The popular telling of this concept is the fact that a butterfly flapping its wings somewhere over the Gulf of Mexico can set off a hurricane that hits New Orleans. In mathematical terms, people have devised toy models such as the Lorenz system for mimicking the dynamics of the Navier–Stokes equations that model the weather, and even the climate. The Lorenz system of equations are nonlinear and chaotic i.e., small changes in input lead to large changes in output. An example that I often give in my course on hyperbolic PDEs is as follows. Consider the Burgers’ equation (another toy PDE model for the Navier–Stokes equations) and consider smooth initial conditions, such as a sinusoidal wave. The input (in this case, the spatial derivative of the initial data) is small; however, the output can be potentially very large, and in fact can even be infinite. This happens because the system is nonlinear. Thus, a sinusoidal wave eventually compresses itself into a shock wave, all in a very short period of time. Similarly, there are regimes where the nonlinearity acts in the opposite direction. We can start with a discontinuous initial data and it rarefacts (or decompresses) to form a smooth output. Thus, the nonlinearity, in this case, transmuted a very large input into a much smaller output. This kind of disproportionate change is the hallmark of nonlinear systems, and is also what makes them very interesting to study.
The long history of numerical analysis has taught us that a first step to design efficient algorithms is to ensure that they are stable
You have studied avalanches in collaboration with the WSL Institute for Snow and Avalanche Research SLF, located in Davos, Switzerland. Also, as an interesting aside, we learn that even the Indian Army is deeply interested in keeping up with the advances in this area. How are avalanches related to fluid flows, if at all?
SM: This is a very interesting question. Let me start by talking about why avalanches are fluid flows. Think of what constitutes an avalanche–-it is a huge and violent moving mass of snow and ice particles. One can think of it as a flow of granular materials, with snow and ice playing the role of granules. Here, to aid your intuition, simply imagine the collective motion of grains of flowing sand. This granular flow can be described in terms of the motion of an incompressible fluid that obeys the law of conservation of momentum. Moreover, the height (depth) of an avalanche is much smaller than both its width and length. Hence, one can apply what is called a shallow fluid approximation, and write down equations that are very similar to the motion of a river, or of even waves in the ocean.
One of the drivers for my interest in avalanches is the collaboration with colleagues at the WSL Institute of Snow and Avalanche Research. The avalanche modelling group there is arguably the best in the world and their simulation software, RAMMS, is really the state of the art in terms of predicting the evolution of avalanches. Interestingly, one of their customers is the Indian Armed Forces, as they need to simulate avalanches to calculate the probabilities of avalanches blocking roads in the Himalayan region–-all of which are of particular importance given the current standoff against China in Ladakh.
Nonlinearity is best encapsulated in how a system responds to inputs
Moving to another problem in Geosciences, your work has also involved the study of tsunamis. How does the idea of “stability’’ in a numerical scheme impact our understanding of real-world phenomena, say in the propagation of a humongously energetic, unusually tall, thick wall of water, as seen in a tsunami?
SM: Tsunamis are again also modelled as shallow-water waves in the deep ocean, as the height of the wave is again, much smaller than its width and length. Thus, one can use variants of shallow flow models, that I had discussed in the context of avalanches, to model tsunamis. In fact, all Tsunami Early Warning Systems (TEWS) employ some version of the shallow-water equations as their core model. These equations need to be discretized in an efficient manner. One of the key issues that comes up in the discretization is to deal with the fact that a tsunami, once triggered by either an earthquake or a landslide, is actually a small perturbation of the underlying steady ocean (the so-called ocean at rest). Since the magnitude of this perturbation can be of the same order as a numerical error, one cannot resolve a tsunami accurately, unless and until one specifically exactly preserves discrete versions of the steady-state of the ocean at rest. Such schemes are termed as well-balanced schemes, and I have contributed to their development in different contexts in the last decade.
Another contribution to tsunami modelling is to deal with the uncertainty of the initial wave height. This wave height is very difficult to measure exactly and can only be modelled probabilistically. Quantifying the resulting uncertainty in wave propagation, in what we term uncertainty quantification (UQ), is therefore of paramount importance in applications; and I have worked quite a bit on algorithms for efficient UQ in tsunamis, with considerable success.
Moving from earthbound flows into outer space, an interesting observation involving the Sun is the mystery surrounding the unusually high temperatures measured, not at the core or even at the surface of the Sun as one would have rightfully expected, but actually at distances millions of kilometers radially away from its core. How has your work in the stability of numerical schemes helped shed light on the problem of high temperatures measured in the Sun’s corona?
SM: The coronal heating problem is one of the outstanding classical problems of astrophysics. There is still no theory that explains this strange phenomenon. A toy version of this problem is the so-called chromospheric heating problem, where the solar chromosphere is at around double the temperature of the photosphere (the visible part of the Sun). I have worked on the chromospheric heating problem, where we built a code to simulate the chromosphere and the lower corona, and observed that waves coming from the interior of the sun, guided by the sun’s strong magnetic field, can carry enough energy to heat the chromosphere, thus providing a plausible explanation for chromospheric heating. The\break key challenge was to find stable discretizations of the underlying MHD equations, which are a formidable coupled system of eight nonlinear equations for plasmas. Nevertheless, we combined different ideas ranging from well-balancing to divergence cleaning, to create a stable discretization framework.
![]() |
![]() |
For most beginning graduate students, it comes as a pleasant surprise that some of the best modern-day approaches to obtaining accurate numerical solutions of otherwise hard nonlinear PDEs, actually owe their success to an ingenious way to interpret and incorporate a grand old idea from thermodynamics, which is entropy. Entropy conservation, dynamics and flux have deeply influenced this entire area of research, resulting in the birth of novel classes of algorithms broadly called Entropy Stable Schemes. Why are these schemes so good at resolving the fine structure of say, colliding shocks inside a turbulent boundary layer–-a typical problem encountered by aerospace engineers?
SM: Entropy plays a very crucial role in the study of hyperbolic systems of conservation laws. The point is that because of the presence of shocks and other discontinuities, one has to seek solutions to these nonlinear PDEs in the sense of distributions. These are weak, non-unique solutions and need to be augmented by additional admissibility criteria, in order to be able to select the physically relevant weak solution. The second law of thermodynamics provides a natural selection criterion, namely that only those weak solutions that are consistent with the second law of thermodynamics are physically relevant, and also have any possibility of being unique. This is indeed true for scalar equations like the Burgers’ equation, and also for the Euler equations in one space dimension.
This is also a good place for me to introduce the concept of well-posedness of solutions to a given PDE. This notion goes back to the French mathematician Jacques Hadamard a century ago, when he postulated that we must seek those solutions of PDEs that satisfy the following three conditions–-solutions must first of all exist (globally in time), and further on must be unique, and finally must be stable with respect to perturbations of inputs. Only if all these three conditions are satisfied, is the underlying PDE then termed as being `well-posed’. Entropy plays a very important role in constraining solutions of nonlinear hyperbolic PDEs, such that they are unique, stable and hence well-posed.
Entropy is also a key constraint in the design of stable numerical schemes. This operates at two levels. First, if the discretization is consistent with a discrete version of the second law of thermodynamics, then we can guarantee that the limit of the numerical approximation, if it exists, is both physically relevant and well-posed in the sense of Hadamard that I discussed just now. Moreover, entropy functions are convex, and controlling the entropy at the discrete level, automatically provides bounds for the approximate solutions in some appropriate function spaces i.e., in square-integrable functions. Thus, an entropy stable scheme is inherently stable, and has a strong chance of correctly approximating a physically relevant solution. The design of entropy stable schemes has a very rich history and can be traced back all the way to Lax and Friedrichs in the early ‘50s. The modern version of entropy stability was formulated by Eitan Tadmor. I started my study of entropy stable numerical methods under the guidance of Eitan and we proved an important result by devising the very first entropy-stable arbitrary high-order finite volume schemes, thus leading to some of the first provable stability properties for high-order schemes for multi-dimensional systems of conservation laws. These results combined two highly desirable properties of numerical approximation, that of stability and accuracy, that I have already alluded to earlier.
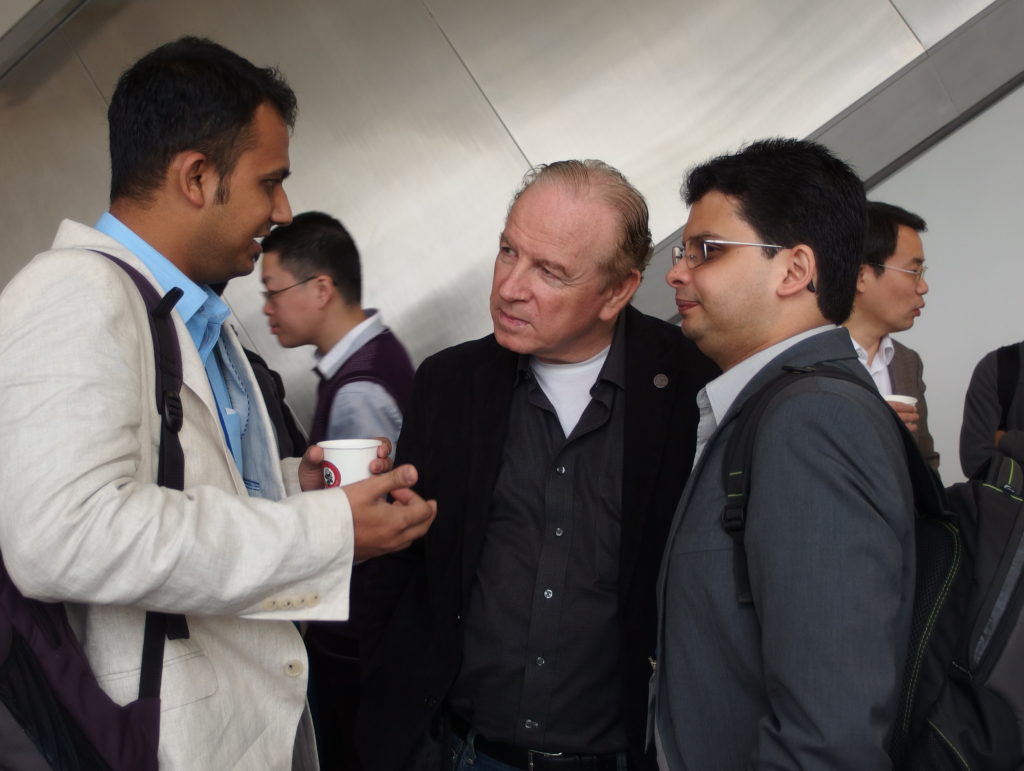
In 2009, in a path-breaking paper published in the venerable Annals of Mathematics, Camillo De Lellis and Laszlo Szekelyhidi Jr. upturned the received wisdom for many decades till then, that entropy solutions were indeed the suitable solution framework for systems of conservation laws in several space dimensions. In particular, they critically questioned the very idea of the uniqueness of such solutions. In the ensuing years, your group along with Eitan Tadmor responded to the challenge by advocating the idea of `Entropy Measure-Valued Solutions’ as the correct framework, and which also guarantees both convergence and uniqueness. Please give us an overview of the rapid developments in this area since 2009 onwards, and on where the subject stands today.
SM: It is true that the conventional wisdom in the field was that entropy solutions are unique, even for multi-dimensional systems of conservation laws; and the fact that we are unable to prove this theorem is solely a limitation of the techniques that we use, and is definitely not conceptual. Nevertheless, very smart people have tried for more than fifty years to find a suitable well-posedness theory for systems of conservation laws in several space dimensions and have failed. As Peter Lax says in his Gibbs lecture in 2007, “There is no theory for the initial value problem for compressible flows in two space dimensions once shocks show up, much less in three space dimensions. This is a scientific scandal and a challenge’’–-this is clearly an outstanding open problem.
As you rightly pointed out, De Lellis and Szekelyhidi struck a blow to conventional wisdom, starting around 2009–10. The genesis of their work goes back to the related problem of incompressible Euler equations, the fundamental governing equations for perfect fluids. Although these equations do not contain shocks, there is no guarantee that the solutions of these equations are smooth, even if the initial data is smooth. This is ascribed to turbulence, characterized as it is by the presence of energetic structures at a very large and diverse number of scales. Thus, a concept of an admissible weak solution i.e., a solution that is of bounded energy has been advocated for these equations, analogous to entropy solutions for systems of conservation laws. Already in the ‘90s, Vladimir Scheffer and Alexander Shnirelman had shown that there could be an infinite number of weak solutions to the incompressible Euler equations. The beauty and depth of the work of De Lellis and Szekelyhidi lay in extending these results to admissible weak solutions. Moreover, by a rather elegant yet simple argument, they were able to construct infinitely many entropy solutions for systems of conservation laws. Their program has been extended in all possible directions now, and they and their collaborators have even proved the so-called Onsager’s Conjecture, and established that there could be infinitely many Hölder continuous solutions of certain physically relevant Hölder exponents.
However, these results are still in a real sense, negative results. They tell us that entropy or admissible solutions are not unique in some cases, but do not necessarily provide any information about what other types of solutions exist, which going further, are also unique. On the other hand, there are good reasons to believe that the Euler equations (both compressible and incompressible) do indeed model the underlying physics. After all, we have been computing the solutions of these equations for more than seventy years with great success. To quote Lax, again from his Gibbs lecture, “Just because we cannot prove that compressible flows with prescribed initial values exist, doesn’t mean that we cannot compute them’’. So, there is a widespread belief that stable numerical methods somehow find the right solutions. My research program is to find out what sort of solutions are the numerical approximations really converging to.
We first identified a framework of solutions, the so-called entropy measure-valued solutions, as a possible candidate. These are no longer functions, but rather space-time parameterized probability measures on phase space. We proved that robust numerical algorithms converge to entropy measure-valued solutions. However, these solutions are inherently non-unique. These objects also do not contain enough desired information and need to be augmented with extra information.
Digging deeper, we arrive at the details of the recent theoretical and conceptual breakthrough that you and your team have effected. And this is the idea of the so-called “Statistical Solutions’’ to otherwise vexing problems, where numerical quantities of interest stubbornly refuse to converge. Kindly walk us through this highly non-obvious and non-trivial set of novel theoretical results that guarantee convergence of numerical algorithms, and also upon which you elaborated in your International Congress of Mathematicians (ICM) 2018 address.
SM: The starting point for statistical solutions was the lack of adequate information in measure-valued solutions that would have constrained the dynamics enough to recover uniqueness. We realized that a reason for this was that measure-valued solutions are point probability distributions and do not contain any information on spatio-temporal correlations. As you can imagine, solutions of PDEs at different points are indeed correlated. So, we need correlations but once we put in two-point correlations, the nonlinearities couple things up and the evolution of these two-point correlations demand us to invoke and describe three-point correlations. This process gets iterated upward, and we end up with having to describe correlations of the solution between countably infinite numbers of points in the domain.
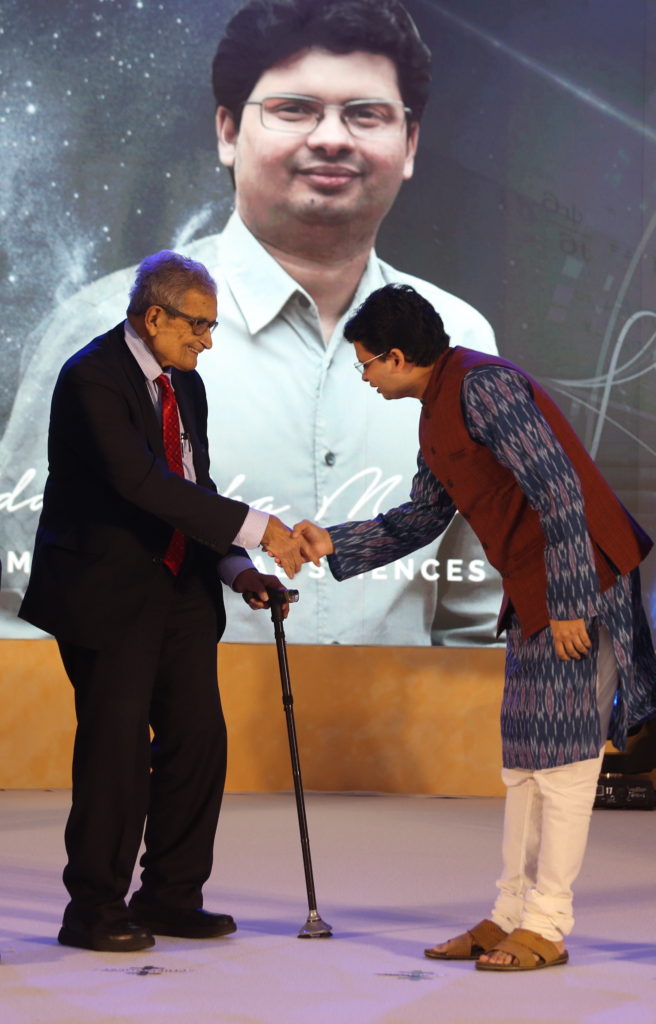
Working with infinitely many correlations can be very difficult technically. Thankfully, we were able to prove a theorem that this infinite family of correlations are totally equivalent to just a single object, i.e. a time-dependent probability measure on spaces of integrable functions. This is the founding basis of statistical solutions. So, statistical solutions are probability measures on infinite dimensional spaces that encode information about the solutions of PDEs. I believe that statistical solutions are really the way forward, and we are excited that we are already able to prove a few partial results in that direction. For instance, we prove under certain hypotheses, that robust numerical algorithms actually converge to statistical solutions. These hypotheses are completely motivated by a variant of the hypotheses used by Andrei Kolmogorov in his 1941 theory of homogeneous isotropic stationary turbulence, and which are also widely believed to hold for real flows. So, statistical solutions are quite promising as a potential solution concept. To quote Kolmogorov, “The epistemological value of probability theory is based on the fact that chance phenomena, considered collectively and on a grand scale, create non-random regularity’’. So, our hope is that a chance phenomenon produces some discernible order at the statistical level, and also provides a unique description of the dynamics.
In the above context, the key role played by an idea known as “Young Measures’’ is clearly seen. This idea, originally due to Lawrence C. Young’s work in the early 1940s, has reappeared later in various contexts via the works of Ron DiPerna and Andrew Majda, Luc Tartar, and David Kinderlehrer–-all in the last eighty years of its existence. Is it possible to physically appreciate the idea of a Young measure, although it may be intrinsically probabilistic in its basic formulation?
SM: I shall try to explain how one could visualize a Young measure. It is easy to imagine a function, say the temperature over Bengaluru at a certain time. Evaluating this function would require us to choose a spot (a space coordinate), say just above the J.N. Tata statue in the IISc campus, and so let us put a thermometer there. This thermometer will provide a unique value of the temperature at that point. However, as we know, every measurement has some intrinsic uncertainty and if you repeat the above measurement, either with a different thermometer, or using the same thermometer but now with temperature readings taken at two different but closely spaced time intervals, you will still record slightly different values. Just try to measure your own body temperature, to see this effect. So, in practice, what we obtain is really a range of values of the temperature, and if we now had access to a lot of measurements, we could plot a histogram that approximates the probability distribution of the temperature. Now, this probability distribution, which depends on which point you measure, and at what time you measure, is indeed a Young measure. If it so happens that there is exactly only one value that always comes up, no matter how many different readings are taken, then the Young measure trivially reduces to a function.
In fact, one can continue this visualization a bit further. Let us imagine the temperature now, but over all of Bengaluru, again at a certain time. This is a single object in a so-called `function space’. Given the previous description of the uncertainty of measurements, we can generate a probability distribution over possible temperatures. This precisely, is now a statistical solution.
Very intuitively put, indeed. In a surprising turn of events, this subject naturally intermeshes with an idea that is of deep and urgent interest to researchers in a wholly different enterprise, which is machine learning. We are referring to ideas surrounding `uncertainty quantification’. In your own work, uncertainty is a quantity that has to be precisely quantified not only at the input initial conditions, but also at the output, which is the set of solutions to the chosen system of conservation laws. How fertile is this unexpected cross-pollination of ideas between these two apparently disparate looking subjects turning out to be?
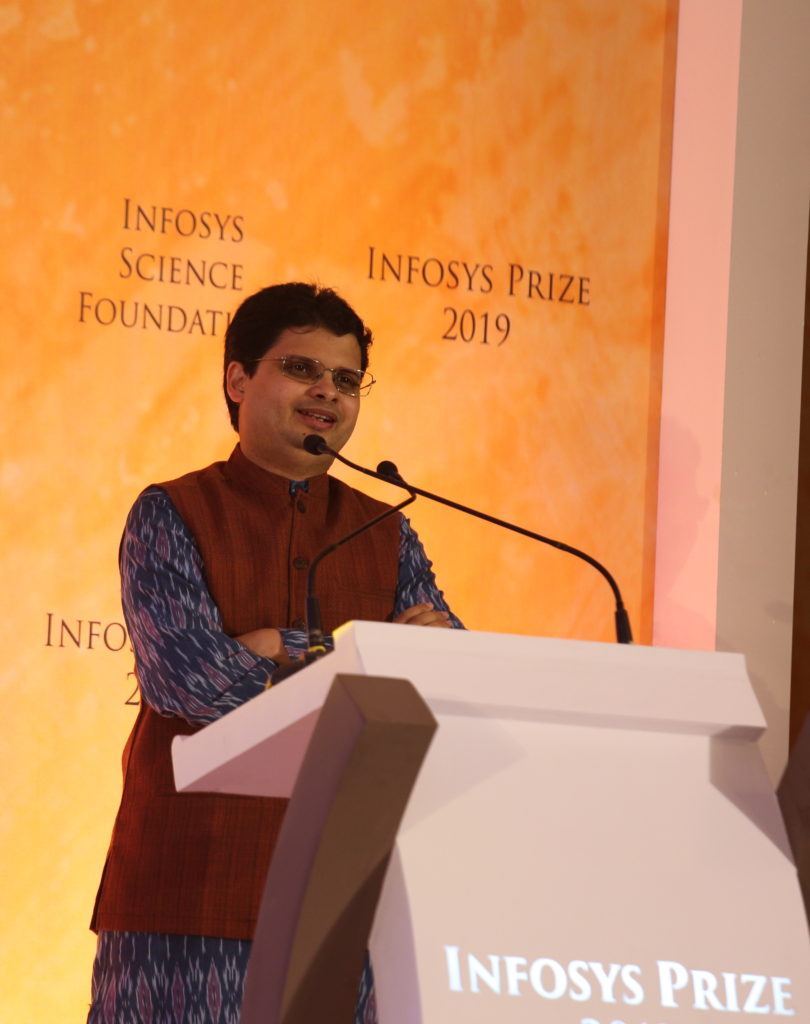
SM: I am glad that you asked me this question about uncertainty quantification and machine learning, as a lot of my current research strongly revolves around these topics. UQ is exactly what I have described before. There are measurement uncertainties in the inputs to the PDE, and these uncertainties propagate into the solution. UQ is the task of computing output uncertainties, given a statistical description of input uncertainties. Naturally, statistical solutions are the right framework for UQ, as both the input (initial data) and output (solution) are probability measures on function spaces.
Currently, the most robust algorithm that we have for computing statistical solutions, and for UQ, is a Monte Carlo type sampling algorithm. It is stable and convergent, but the rate of convergence is slow. Hence, it can be very expensive. In fact, a single UQ computation for a three-dimensional fluid flow requires possibly thousands of node hours of crunching, and that too on leadership-class supercomputers. Hence, there is a pressing need for coming up with faster and cheaper algorithms. In this context, we hope to employ machine learning algorithms. We have already done so for many examples of fluid flows within the paradigm of what one calls supervised learning, but the fully turbulent regime is still an open problem.
Independently, I use different paradigms in machine learning to design fast algorithms for PDEs. This program has already had some success and we expect to push this line of research to a far greater extent in the coming years. I envisage machine learning algorithms possibly even replacing traditional numerical methods for PDEs in many contexts.
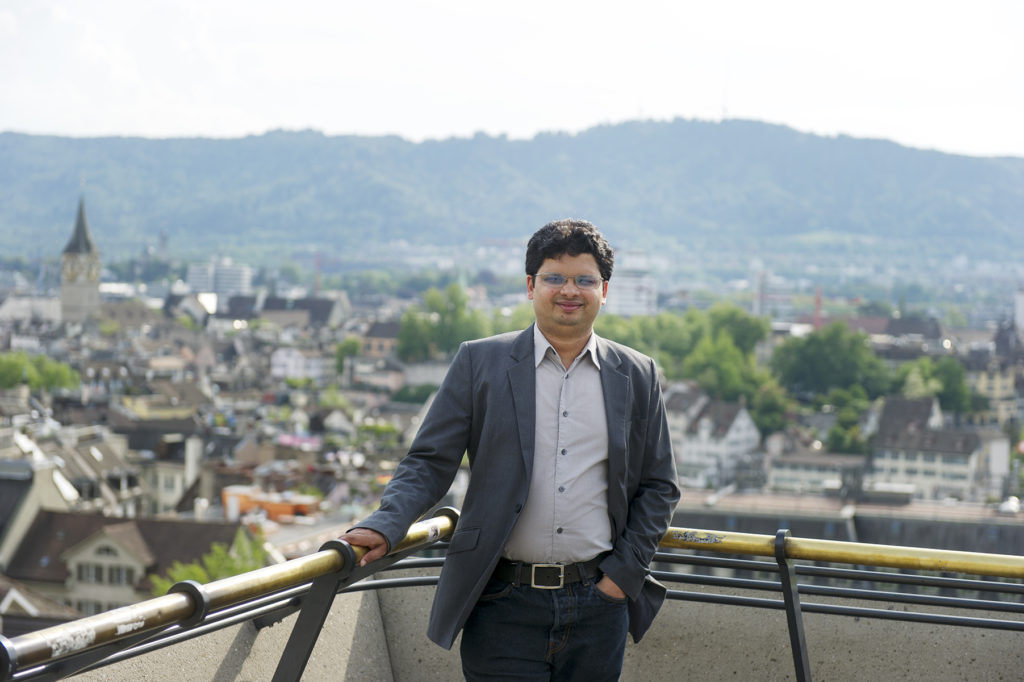
In the last ten years since the De Lellis and Szekelyhidi Jr. paper arrived, the subject of the theoretical foundations of fluid dynamics has seen not only an explosion of ideas but also the springing up of exceptional new talent. Your own work, along with those of Tristan Buckmaster, Vlad Vicol and Phil Isett, has again revitalized this timeless subject. As a member of this young and talented brigade charting new territory in the study of the flow of fluids, what do you see as key milestones in the journey ahead, and also, what really are the specific reasons for this huge current excitement in the area of theoretical and computational fluid dynamics?
SM: There are many reasons to be optimistic about theoretical and computational fluid dynamics at the moment as many new results are coming to light, in what is actually considered to be a very mature area–-even though the central problems themselves remain unresolved. On the analysis side, you have the whole school of convex integration, which has obtained outstanding results about the lack of uniqueness of even reasonably regular (Hölder continuous) solutions for the fundamental equations of fluid dynamics; and they keep on extending the envelope of PDEs, the well-posedness of whose solutions is brought into question by these results. This program casts serious doubts about the very applicability of the fundamental equations of fluid dynamics as we know them today, in order to describe natural phenomena.
On the other hand, our group and a few others argue that statistical concepts are the key to understanding the role of the fundamental equations of fluid dynamics, with the subtext that there is not much wrong with the basic models themselves, but rather only with their interpretation. Of course, we have a long way to go in order to produce the correct constraints on solutions, and rigorously establish some sort of well-posedness. However, this approach has a possible path towards building a theory that explains the role of the fundamental equations of fluid dynamics.
On the computational side, we are increasingly able to solve large-scale problems, for instance in UQ and also Bayesian inverse problems. This trend will be further accentuated by the emergence of machine learning algorithms in computational fluid dynamics (CFD). So, there are plenty of reasons to be excited about what the future holds.
Your work has been recognized by the International Council for Industrial and Applied Mathematics (ICIAM) with the awarding of the Collatz Prize for the year 2019. In 2015, even before you turned thirty six, you were awarded the Richard von Mises Prize. You were also an Invited Speaker in the ICM held in Brazil in 2018, recognizing your pioneering contributions. What do you personally feel are the most important questions that need to be addressed at this juncture, given the wealth of recently accumulated new knowledge?
SM: I have been really honoured by these awards and recognitions. An enormous amount of credit goes to my collaborators, particularly my PhD students and postdocs whose contributions were pivotal. My family has also been a great source of support all these years.
The problems that my group is working on currently includes further investigation of the mathematical and computational aspects of statistical solutions of the fundamental equations of fluid dynamics. We believe that this approach will lead to new insights into these hitherto, hard to tackle problems. My group is also actively working on adapting machine learning algorithms to solve PDEs. I think these new algorithms offer the promise of becoming a game-changer in the area of scientific computing.
Dear Professor Siddhartha Mishra, it has been an absolute pleasure talking to you about your passion, and walking along with you in what has so far been a fascinating journey. On behalf of our team, I extend to you again the warmest of wishes, and look forward to many more interactions in the future.
SM: Thank you!
acknowledgement The author wishes to thank Deep Ray, a former joint PhD student of Siddhartha Mishra, alongwith Ms.Veena and Mr. P Desaiah of TIFR CAM, Bengaluru for help in sourcing some of the photos used in the article.\blacksquare